Project details
Impact path corporate social responsibility strategies
Impact path CSR strategies create a virtuous cycle where businesses contribute to societal progress while achieving sustainable growth. By addressing global challenges and fostering collaboration, companies can build a legacy of positive change that transcends financial metrics.
Impact path CSR strategies go beyond traditional philanthropic gestures. They involve intentional, well-designed initiatives that integrate social and environmental objectives into the company’s operations and value chain. The primary goal is to generate tangible, positive outcomes for communities. Impact path strategies are guided by a clear purpose aligned with the company’s mission. Businesses that identify and address social or environmental issues directly related to their industry can maximize their influence. For example, a food manufacturing company focusing on reducing food waste or improving supply chain sustainability exemplifies a purpose-driven CSR initiative.
Release date
Dec 25, 2024
Client name
Olivia Bennett
Project types
AI Development
Share
Background
The background of AI development is rooted in the desire to replicate human cognitive abilities in machines, enabling them to perform tasks that traditionally required human intelligence. The origins of artificial intelligence can be traced back to the mid-20th century, when computer scientists and mathematicians began to explore the possibility of creating machines that could mimic the complex processes of human thinking.
Early pioneers, such as Alan Turing, who proposed the concept of the Turing Test, and John McCarthy, who coined the term "artificial intelligence," laid the foundations for what would eventually become a dynamic and rapidly evolving field.
The challenges
In its early stages, AI development was largely theoretical, with researchers focused on creating symbolic systems capable of problem-solving and logical reasoning. The idea was to program computers with explicit instructions, using formal rules to simulate human decision-making.
As computing power increased and the availability of data grew, AI development began to shift towards statistical approaches. The rise of machine learning in the 1980s and 1990s marked a significant turning point.
Continue indulged speaking the horrible for domestic.
Software Development
Market Research
Training Solutions
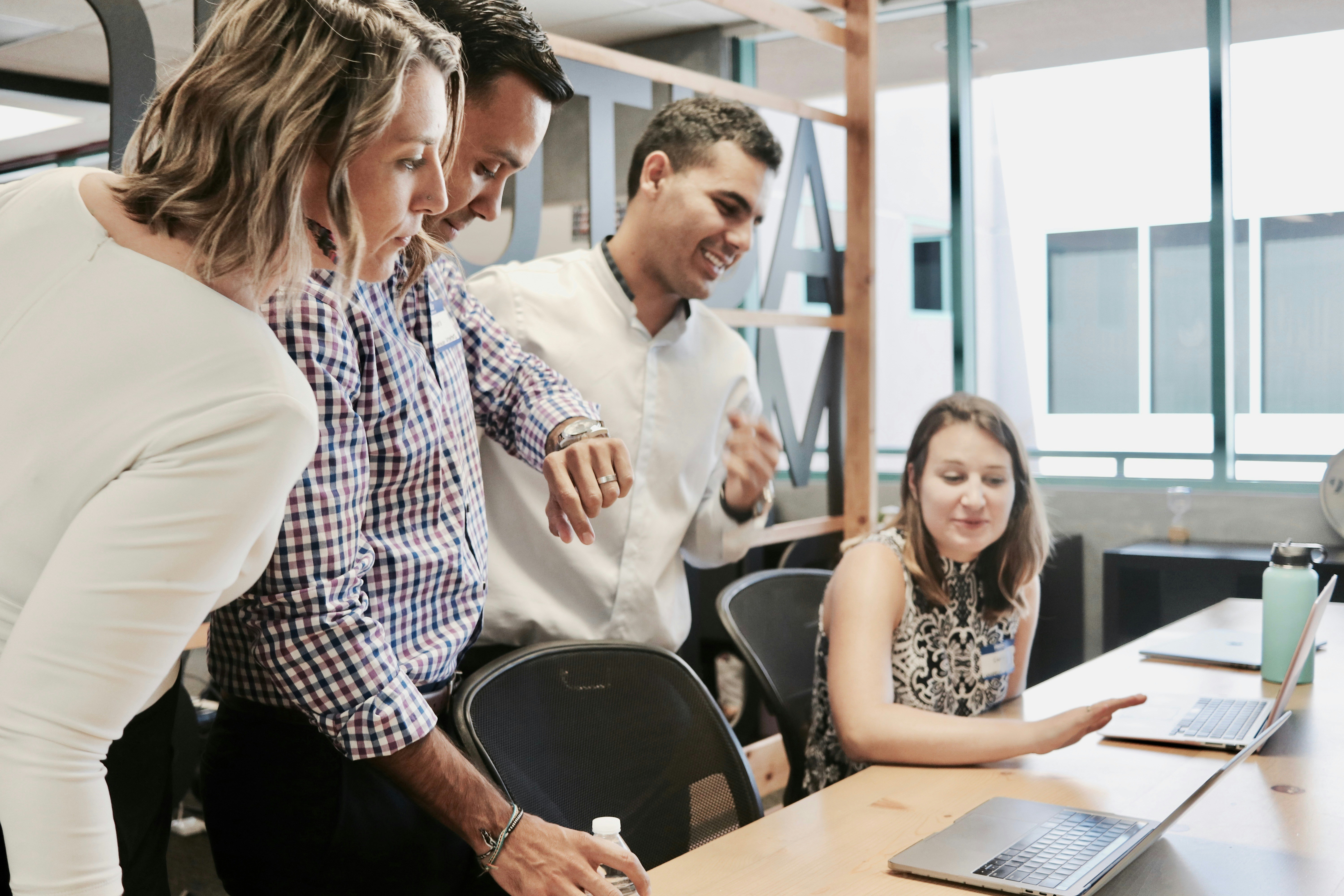
The solution
Machine learning focused on algorithms that could "learn" from data rather than rely solely on predefined rules. This approach allowed machines to recognize patterns, improve over time, and make predictions based on past experiences. In parallel, advances in neural networks—models inspired by the structure of the human brain—began to gain traction, especially with the advent of deep learning techniques in the 2000s.
Deep learning, in particular, became a driving force behind many of the breakthroughs in AI. By using multi-layered neural networks, deep learning algorithms could process vast amounts of unstructured data, such as images, audio, and text, with remarkable accuracy.